Understanding Spam Filter Machine Learning for Business Enhancement
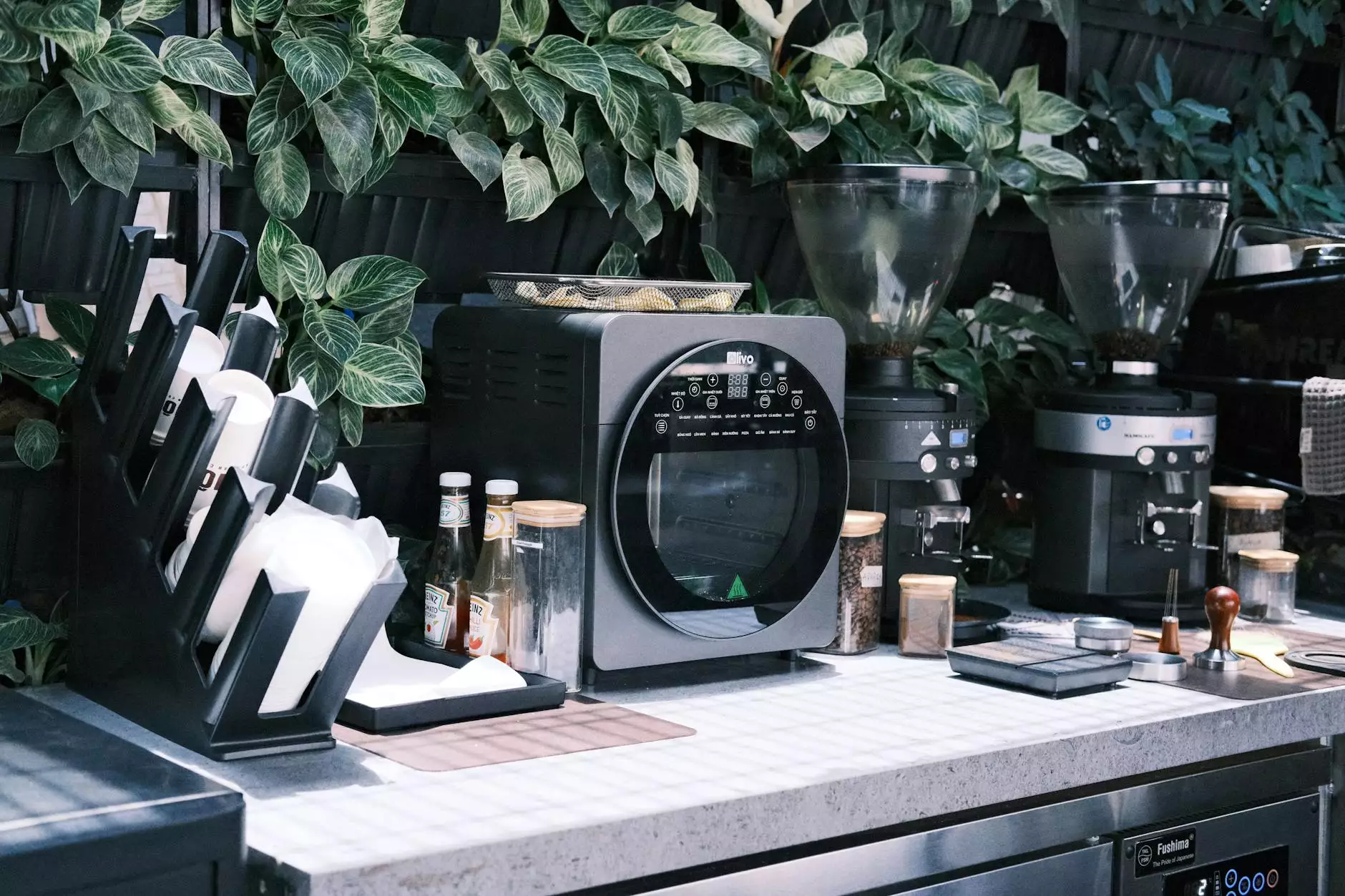
In today's digital era, the emergence of spam filter machine learning has revolutionized the way businesses operate, especially in the fields of IT services and security systems. Companies like spambrella.com are at the forefront of integrating machine learning algorithms to create robust spam filtering solutions that not only protect sensitive data but also optimize operational efficiency. This article delves deep into the intricacies of machine learning in spam filtering and how it empowers businesses.
What is Machine Learning in Spam Filtering?
Machine learning (ML) is a subset of artificial intelligence (AI) that enables systems to learn from data and improve over time without being explicitly programmed. When applied to spam filtering, machine learning involves the use of algorithms to analyze vast amounts of email data and identify patterns that categorize emails into 'spam' or 'not spam'. This intelligent classification reduces the chances of invaluable emails being lost amidst unwanted noise.
How Does Spam Filter Machine Learning Work?
Spam filters utilize various machine learning techniques, the most common of which include:
- Supervised Learning: In this approach, the algorithm is trained on a labeled dataset, where emails are pre-classified as spam or non-spam. The model learns to identify elements that distinguish spam from legitimate emails.
- Unsupervised Learning: Here, the algorithm analyzes a set of emails without pre-existing labels. It identifies patterns and clusters in the data, which can indicate spam-like behavior.
- Reinforcement Learning: In this technique, the model learns to make decisions by receiving feedback from its actions—classifying an email as spam or not—allowing it to continuously improve its accuracy.
The Importance of Spam Filter Machine Learning for Businesses
The integration of spam filter machine learning into business practices presents several compelling advantages:
1. Enhanced Security
Protecting sensitive information is crucial for any organization. The application of machine learning in spam filtering significantly reduces the risk of phishing attacks and malware infiltrating business communication channels. By continually adapting to new threats, these smart filters provide an evolving defense mechanism against cyber threats.
2. Increased Productivity
Employees spend a considerable amount of time managing their emails. Effective spam filters help in minimizing the distractions caused by unwanted emails, allowing employees to focus on value-added tasks. This increased productivity contributes to better overall performance within the organization.
3. Cost Efficiency
Investing in spam filter machine learning solutions can lead to substantial cost savings. By preventing fraudulent emails and the potential fallout from data breaches, companies can avoid the hefty financial consequences associated with cyber incidents. Moreover, decreased time spent managing spam translates into reduced labor costs.
4. Improved Customer Satisfaction
A well-functioning spam filter ensures that important communications from customers are not lost in spam folders. By improving the flow of information, businesses can enhance their customer service, leading to better relationships and increased loyalty.
Best Practices for Implementing Spam Filter Machine Learning
1. Choose the Right Machine Learning Model
Different spam filtering scenarios may necessitate different machine learning models. Businesses should evaluate their needs and select models that best fit their unique requirements, whether they opt for supervised, unsupervised, or reinforcement learning methodologies.
2. Regularly Update Data Sets
The effectiveness of machine learning algorithms hinges on the quality and recency of their training data. Regularly updating the datasets with new examples of both spam and legitimate emails ensures that the model remains robust against emerging threats.
3. Continuous Monitoring and Feedback Loops
Implementing continuous monitoring mechanisms allows businesses to refine their spam filters based on real-world performance. Establishing feedback loops where users can flag misclassified emails aids in improving the system's accuracy over time.
4. Foster User Awareness and Training
While machine learning significantly enhances spam filtering capabilities, user awareness remains crucial. Conducting training sessions on identifying phishing attempts and social engineering tactics will empower employees to act prudently when navigating their email environments.
Real-World Applications of Spam Filter Machine Learning
Many organizations have successfully adopted spam filter machine learning, leading to transformative changes in their email management and security processes. Here are some notable applications:
1. Financial Institutions
Financial organizations handle vast quantities of sensitive customer data, making them prime targets for spam and phishing attacks. By deploying machine learning-based spam filters, these institutions can safeguard customer information and maintain cybersecurity compliance.
2. E-commerce Platforms
E-commerce businesses rely heavily on email marketing and communication with customers. Spam filter machine learning ensures that marketing emails reach their intended audience while unwanted spam is filtered out, thereby optimizing conversion rates and maintaining a clean email list.
3. Healthcare Providers
Healthcare organizations must prioritize patient confidentiality while simultaneously managing numerous emails daily. Advanced spam filtering technologies allow providers to streamline patient communications safely and effectively.
Future Trends in Spam Filtering Technology
The landscape of spam filtering is ever-evolving, with promising trends on the horizon:
1. AI and Deep Learning Integration
As AI technology progresses, deep learning models are expected to revolutionize spam filtering through more comprehensive pattern recognition capability. These advancements will allow systems to discern subtler distinctions between spam and legitimate emails.
2. Predictive Analytics
Predictive analytics can be harnessed to anticipate emerging spam trends based on historical data. By understanding how spammers evolve their tactics, organizations can proactively adjust their filtering strategies to counteract these new methods.
3. Collaborative Filtering
Collaborative filtering involves sharing insights from multiple organizations regarding spam trends. By pooling data, businesses can improve their spam filters’ accuracy and learn from the experiences of others.
4. User-Centric Approaches
Future spam filtering solutions will likely prioritize user inputs and preferences, leading to more personalized experiences. Filters that adapt to each user’s specific behavior and preferences will significantly enhance overall user satisfaction.
Conclusion
In conclusion, the advent of spam filter machine learning has brought about significant enhancements in how businesses manage email security and IT services. From improving operational efficiency to safeguarding sensitive information, the benefits are manifold. As businesses increasingly recognize the importance of robust spam filters, the ongoing evolution of machine learning technologies will undoubtedly lead to even more sophisticated solutions. By adopting best practices in spam filtering and remaining vigilant against evolving threats, organizations can ensure a secure and productive future.
For more details on implementing advanced spam filtering solutions, visit spambrella.com today!