Enhancing Business Efficiency through Data Annotation
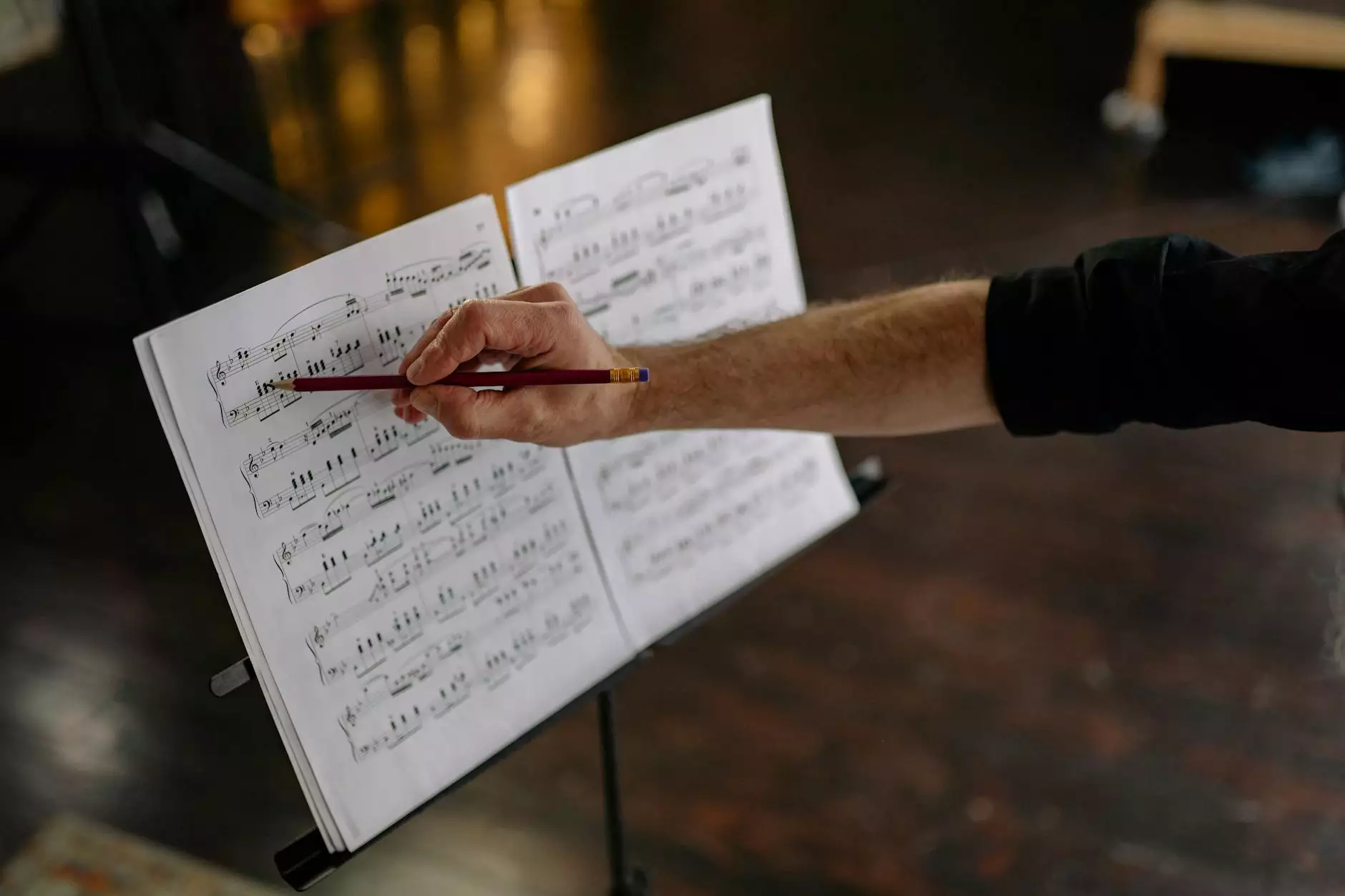
In today’s fast-paced digital landscape, businesses continually seek methods to enhance their operations and decision-making processes. One of the essential strategies is to annotate datasets, a crucial step that can significantly improve a company's ability to analyze information and make informed choices. This article will not only explain the concept of data annotation but also delve into its applications, benefits, and how it can specifically serve industries like home services and locksmithing.
Understanding Data Annotation
Data annotation involves the process of labeling or tagging data to provide context and meaning. This is critical for training machine learning models, particularly in processes like natural language processing (NLP) and computer vision. In simpler terms, it’s how we make datasets understandable and usable for algorithms that drive insights.
Types of Data Annotation
- Image Annotation: This includes drawing bounding boxes around objects in images, which aids in visual recognition tasks.
- Text Annotation: This involves marking up text to provide information such as part-of-speech tagging or entity recognition.
- Video Annotation: Here, frames of video are annotated to identify motion or actions within the scenes.
- Audio Annotation: This type focuses on labeling audio files for tasks like speech recognition.
The Importance of Annotating Datasets in Business
For businesses, particularly those operating in fields like home services and keys and locksmiths, the ability to use data effectively is paramount. By annotating datasets, companies can unlock insights that drive success. Let’s explore why this practice is so important:
Enhancing Decision-Making
The primary advantage of data annotation is improved decision-making. By transforming raw data into understandable formats, businesses can analyze trends and patterns more efficiently. For example, a locksmith company tracking customer feedback can annotate sentiment within reviews to adjust services accordingly.
Training Machine Learning Models
Machine learning relies heavily on annotated data. In home services, machine learning models can predict when a household might require maintenance. By using historical datasets annotated with repair history and customer behavior, companies can forecast issues before they arise, leading to proactive service offerings.
Improving Customer Experience
When businesses annotate datasets related to customers, they can better understand and anticipate customer needs. This can lead to personalized marketing strategies. For instance, a locksmith service could use annotated data to send targeted promotions to customers who have recently moved or may require new security installations.
How to Effectively Annotate Datasets
Now that we understand the significance of data annotation, let’s discuss how businesses can implement it effectively.
1. Define Clear Objectives
Before embarking on the annotation journey, it’s crucial to define what the goals are. What insights are you hoping to gather from the data? For a home services business, this could involve understanding seasonal trends in service requests.
2. Choose the Right Tools
There are various tools available for data annotation. Options range from manual annotation tools to automated software. It’s essential to select tools that fit your specific business needs and budget. For locksmith businesses, tools that enable quick tagging of customer feedback might be necessary to keep pace with service demands.
3. Ensure Annotation Quality
The accuracy of data annotation directly impacts the effectiveness of the resulting analysis. Implementing a quality control process is crucial. This could include regular reviews of annotated data and providing continuous feedback to annotators.
4. Collaborate with Experts
If data annotation falls outside your team's expertise, consider partnering with specialists. Companies like keymakr.com can support businesses by providing high-quality data annotation tailored specifically for industries like locksmithing and home services, ensuring the accuracy and relevance of annotations.
Best Practices for Annotating Datasets
Following best practices can enhance the effectiveness of your annotation processes:
1. Develop a Comprehensive Annotation Guide
An annotation guide should outline the standards and protocols for annotators to follow, ensuring consistency across the dataset. This guide is especially useful in industries where specific terminologies and classifications are common.
2. Utilize a Sample Dataset
Before annotating the entire dataset, it’s advisable to conduct a trial run with a smaller sample. This practice allows you to identify challenges and adjust methods accordingly, ensuring a smoother process when working with larger datasets.
3. Focus on Contextual Relevance
When annotating data, it's essential to consider the context in which it will be used. For example, if you’re annotating customer interactions, understanding seasonal fluctuations can guide how you categorize and label this information.
4. Continuously Improve Your Process
The business landscape is always changing, and so should your data annotation processes. Regularly assess and refine your methodologies based on performance metrics and feedback from stakeholders to ensure your approach meets evolving needs.
Case Studies: Data Annotation in Action for Home Services and Locksmiths
To illustrate the power of annotating datasets, consider the following case studies:
Case Study 1: A Local Locksmith
A local locksmith implemented a machine learning model that predicted peak service times based on historical booking data. By annotating past service requests with data such as time, type of service required, and customer demographics, they developed an efficient schedule ultimately increasing service capacity by 30% during peak times.
Case Study 2: Home Service Maintenance Company
A home services company that provided diverse services from plumbing to electrical repairs began annotating customer feedback. By tagging specific words related to service quality, response times, and satisfaction levels, they implemented improvements that resulted in a 25% increase in customer satisfaction ratings in just one year.
Future Trends in Data Annotation
The field of data annotation is constantly evolving, influenced by advancements in technology and consumer demand. Here are some anticipated trends that could shape the future:
1. Automation and AI-Driven Solutions
As AI technology advances, more businesses are expected to adopt automated data annotation solutions. These tools can significantly reduce the time and effort required for data preparation while maintaining accuracy.
2. Increased Focus on Data Privacy
With growing concerns regarding data privacy, businesses need to be diligent about how they annotate datasets. Implementing strict data protection measures during the annotation process will become increasingly important.
3. Integration of Collaborative Annotation Platforms
Collaborative platforms that allow teams to work together on data annotation are expected to gain popularity. These platforms can streamline the process and improve quality through collective insights and expertise.
Conclusion
In conclusion, the process to annotate datasets is not merely an operational task, but a strategic asset that can dramatically elevate a business's capability to make data-driven decisions. For companies in the realms of home services and locksmithing, embracing data annotation can lead to enhanced customer experiences, informed business strategies, and a significant competitive edge. By understanding its importance, implementing effective strategies, and staying abreast of industry trends, businesses can harness the true power of their data.