Maximizing Efficiency with a Machine Learning Labeling Tool
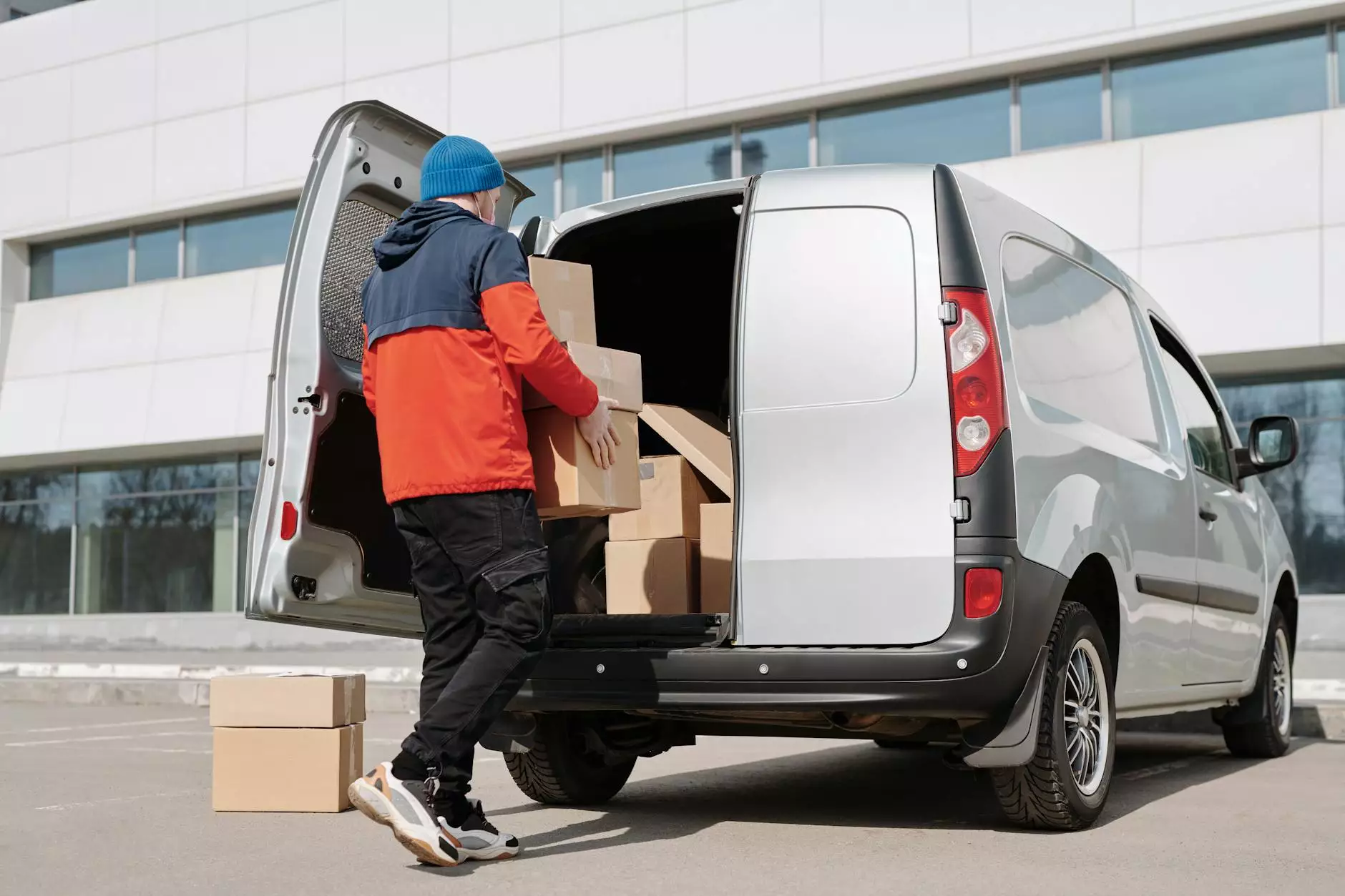
In today's digital era, businesses are increasingly relying on data to drive their strategies and decisions. One of the most significant aspects of leveraging data is the ability to properly label and annotate it for machine learning purposes. This is where a machine learning labeling tool becomes essential. In this comprehensive article, we will take an in-depth look at data annotation tools, their importance, and how utilizing a machine learning labeling tool can transform your data annotation platform into a powerhouse of efficiency.
Understanding Data Annotation
Data annotation is the process of labeling data sets in a manner that can be understood by machine learning algorithms. This typically involves tagging images, text, audio, or video data to help machines recognize patterns and make decisions based on the information provided. As AI continues to evolve, the demand for properly annotated data has skyrocketed.
The Role of Data Annotation Tools
Data annotation tools are software applications designed to aid users in the annotation process. They streamline workflows, ensure consistency, and enhance the overall quality of the annotated data. Some key functionalities of these tools include:
- Customizable Interfaces: Users can tailor the tool's interface to align with specific project requirements.
- Collaboration Features: Allowing multiple users to work together on tagging data ensures efficiency and reduces time to completion.
- Integration Capabilities: Seamless integration with existing systems and platforms can enhance operational effectiveness.
- Quality Control Mechanisms: Tools often include features for reviewing and verifying the accuracy of annotations.
The Importance of a Machine Learning Labeling Tool
When it comes to data annotation, the use of a machine learning labeling tool can significantly elevate the entire process. Here are several reasons why these tools are critical:
1. Improved Accuracy and Consistency
Machine learning labeling tools leverage algorithms that ensure annotations are done with high precision. They can learn from previous labeling tasks, thus improving the accuracy over time. This consistent performance is crucial for producing reliable datasets that enhance the accuracy of machine learning models.
2. Increased Efficiency
Annotating large datasets manually can be a time-consuming process. A machine learning labeling tool automates many of the repetitive tasks involved in data labeling, drastically reducing the time needed to prepare data for machine learning projects. Faster annotation means quicker model training and deployment.
3. Scalability
As businesses grow, so does the volume of data they handle. A robust labeling tool can scale effortlessly, allowing organizations to manage increasing data loads without compromising on quality. This scalability makes it easier for businesses to adapt to changing data needs.
4. Cost-Effectiveness
By automating the labeling process, businesses can significantly reduce labor costs associated with data annotation. Additionally, the improvements in speed and accuracy mean that companies can spend less time fixing mislabeled data, further cutting expenses.
Key Features of a Cutting-Edge Machine Learning Labeling Tool
Investing in a high-quality machine learning labeling tool comes with numerous features that streamline the annotation process. Here are some essential characteristics to look for:
1. Multiple Annotation Types
A sophisticated labeling tool supports various types of annotations, including:
- Image Segmentation: Dividing an image into segments for detailed labeling.
- Object Detection: Identifying and labeling objects within images or video frames.
- Text Annotation: Tagging parts of text for natural language processing tasks.
- Audio and Video Annotation: Labeling sounds and movements in multimedia files.
2. User-Friendly Interface
The user experience plays a vital role in the effectiveness of any tool. A well-designed interface with intuitive navigation ensures that users can focus on annotating data rather than wasting time figuring out how to use the tool.
3. Advanced Collaboration Tools
In a corporate environment, collaboration among teams is crucial. Features that allow multiple users to work on projects simultaneously, along with chat and comment functionalities, make it easier for teams to provide feedback and make decisions swiftly.
4. Machine Learning Integration
By incorporating machine learning capabilities, the tool can suggest labels based on existing data, thereby enhancing user productivity. As users provide more labeled data, the software continues to refine its suggestions, thus improving the overall output.
Choosing the Right Data Annotation Tool
With numerous options available in the market, selecting the right data annotation tool can be daunting. Here are some factors to consider:
1. Specific Use Cases
Identify the types of projects you will need the tool for. Different tools may excel in specific types of data annotation, so ensure that the selected tool aligns with your business requirements.
2. Customization Options
Look for tools that offer customizable features for tailored processes that fit your business's unique workflow.
3. Support and Documentation
Inquire about the level of customer support offered by the vendor. Comprehensive documentation and support resources are essential for resolving any issues that may arise during operation.
4. Pricing Structure
Evaluate the pricing model to ensure it fits within your budget while providing the features you require. Consider both the initial costs and the long-term value offered by the service.
Case Study: KeyLabs.ai
KeyLabs.ai exemplifies a robust data annotation platform equipped with a state-of-the-art machine learning labeling tool. By harnessing advanced features such as user collaboration, customizable interfaces, and extensive support for multiple annotation types, KeyLabs.ai has established itself as a front-runner in the data annotation industry.
Organizations that have utilized KeyLabs.ai report significant reductions in time spent on data labeling, allowing them to allocate resources to more strategic initiatives. The ability to scale effortlessly as data needs grow further solidifies its competitive advantage.
Conclusion: Elevate Your Business with the Right Tool
The future of machine learning and artificial intelligence hinges on the availability of high-quality annotated data. A reliable machine learning labeling tool is not just an asset; it is a necessity for any organization looking to thrive in the data-driven landscape. By investing in efficient and accurate data annotation solutions, businesses can enhance their operational effectiveness, reduce costs, and ultimately, drive better outcomes.
In conclusion, whether your organization is in its early stages or looking to improve existing processes, adopting a machine learning labeling tool is a strategic move that can lead to exponential growth and success. With the right tool, your data annotation platform can unlock the potential of machine learning and set your business on a path to data excellence.
For more information about data annotation tools and services, visit keylabs.ai.